Learning and Employment Records (LERs) will become the primary means for documenting and signaling capabilities, particularly for talent transactions (i.e., enrolling, enlisting, employing). LERs will emerge in ecosystems where learners, credentialing institutions, and LER recipients all find value in the security, portability, and reliability of the information exchanged.
The landscape analysis of emerging LER ecosystems yields four observations:
- Validating Diverse Learning Experiences: An effective credentialing and LER ecosystem acknowledges that learning happens in various settings beyond traditional classrooms. A wide variety of learning experiences are valuable and should be a part of an LER.
- AI-Powered Skill Identification and Competency Mapping: AI will play a crucial role in analyzing learning experiences and associated evidence. The AI tools will be uniquely capable at extracting, organizing and then storing skills developed and demonstrated within each experience.
- Moving from Skills to Credentials: Credentialed skills will be the core currency of employment and admissions, while verified experiences will be the building blocks and leading evidence of context and skills applied.
- Building a Competency Profile: For skills credentials to be valuable, a learner’s competency profile needs evidence of the applied skills (experiences) and should have a variable rating system to account for the provided evidence and accommodate a range of experiences.
A Taxonomy for Experience Credentialing
An effective credentialing and LER ecosystem acknowledges that learning happens in various settings in and beyond traditional classrooms. A variety of quality learning experiences are valuable, not well represented by skill credentials, not verifiable in a resume and, as a result, should be a part of an LER.
These valuable learning experience categories include:
- Employment: Work experience where the learner is paid.
- Work-Based Experiences: Apprenticeships, internships, client-connected projects.
- Entrepreneurial Experiences: Learner-identified opportunity designing solutions and delivering value to a community, such as starting a business to social impact projects.
- Civic Engagement: Participation in civic/community organizations and political activities.
- Service: Participation in addressing a community need (typically identified by someone else).
- Independent: Independent learning experience consists of inquiry (science fair), adventure (travel) and expression (art, music, language). These are typically self-directed experiences.
- Course or Class: (high school or college credit or certificate): Completing courses will continue to be a valuable way to develop skills and competencies.
- Extra-curricular: These experiences include participation and awards in sports, speech and debate, clubs, drama, etc.
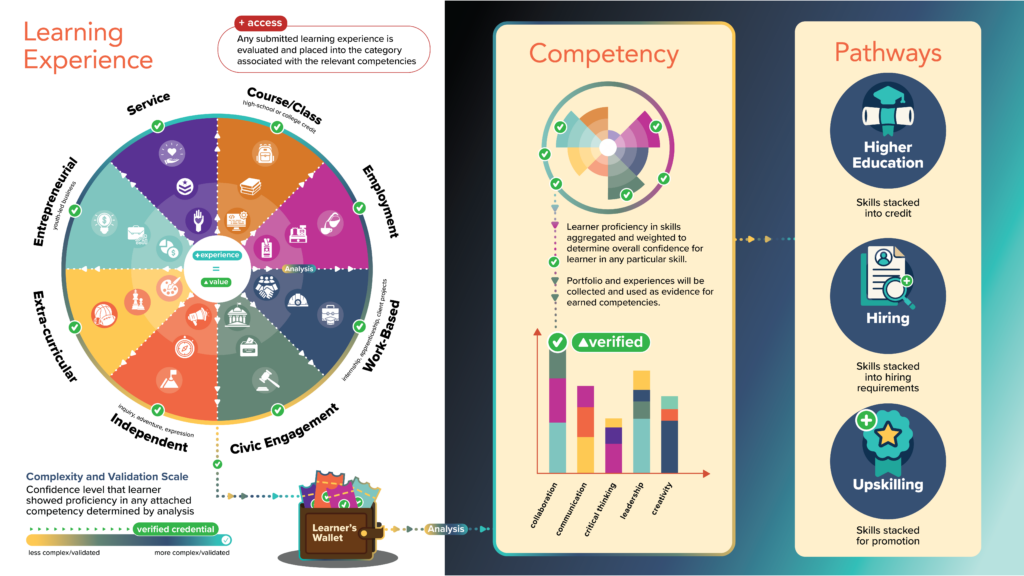
A learner’s life experiences would be submitted and then stored in their LER. In each instance, the submitted experience and corresponding evidence (portfolios, multimedia artifacts, reflective dialogue (e.g., SchoolJoy) and qualitative/quantitative entry) would be weighed on a low to high confidence scale. For example, a learner who submits an experience (and evidence) of starting a non-profit in high school to provide blankets to housing insecure community members will have a higher confidence rating in certain durable skills than someone who submits a similar experience with fewer pieces of evidence or a smaller scope of project (e.g., participating in a one-hour service learning experience serving food to housing insecure community members). These experiences would be verified using endorsements from supervisors, portfolios, qualitative entries, etc. using Experience Quality Indicators (EQIs).
- Responsibility: What was your role in the experience? How available were resources, mentorship and collaboration?
- Complexity: How difficult and intricate was the experience? How long was the experience?
- Novelty: Who created the experience? Whose idea was it?
Additionally, these experiences would supply metadata such as sector, skills (core, technical, durable), duration, org type, geo, etc., to support the verification process and communication with Higher Ed and employers.
Experience Quality Indicators
Category | Level 1 (Low) | Level 2 | Level 3 | Level 4 (High) |
Responsibility | Minimal involvement; completed tasks as directed by others. No need for resource management or collaboration. | Some responsibility; contributed to tasks but relied on guidance from others. Limited use of resources and occasional collaboration. | Significant responsibility; took initiative in some areas, actively sought resources and engaged in collaborative efforts. | Full responsibility; led the experience, actively managed resources, sought mentorship, and facilitated extensive collaboration. |
Complexity | Simple tasks; required little skill and were completed in a short time frame. | Moderately challenging; involved a few complex elements but manageable with some effort over a moderate time period. | Complex; involved multiple challenging elements requiring problem-solving and sustained effort over an extended period. | Highly complex; involved intricate, multi-layered challenges, required advanced skills, and extended over a significant duration. |
Novelty | Followed an existing framework or idea created by others. No original input. | Adapted an existing idea with minor modifications; some elements were personalized but largely followed established practices. | Created a new approach or significantly modified an existing concept; demonstrated creativity in its execution. | Fully self-initiated; originated and designed an entirely new experience from scratch, showcasing high innovation. |
Learners could have varying levels of depth and verification in each experience, but students would be encouraged to participate in a certain number of diverse experiences within each category. These diverse learning experiences merge multiple skills, drive learning in the real world and challenge students while building critical assets like social capital and networks, knowledge of possible career pathways and more. These experiences also could serve as multipliers for achieving competency more rapidly.
The submitted information would then be interpreted and translated by artificial intelligence (AI) tools into a discrete set of high-value skills as indicated by employers, higher education and K-12.
Over time, these cumulative skills demonstrations would stack into competencies at varying rates (depending on quality indicators and validation) that have value for higher education admission, employment or upskilling/promotion with current employers. A learner may have achieved competency in Creativity, but based on the evidence, the algorithm may indicate low confidence in the ability to perform the competency across multiple contexts. This vetting of confidence would help both employers/recruiters and higher education institutions derive a sliding scale of value from the competency. These competencies, backed by evidence (experiences) and a confidence rating (verifiability), would then become the building blocks for earning credentials in the high-value skills. In the end, both competencies and experiences have value in higher education, employability and promotion and thus are credentials.
As this method is geared to capture all experiences, it will require interoperability with a wide variety of experience types and also assessment types (mentioned earlier).
Scenario: Entrepreneurial Experience – Starting a Non-profit
Experience Description: A student named Maria is participating in ProX, a platform used by Real World Learning participating districts. She is paired with an entrepreneurial mentor and decides to start a nonprofit organization that provides free coding lessons to underserved youth in their community. Maria organizes a team, secures funding through grants, and collaborates with local schools to host the sessions.
Experience Quality Indicators Evaluation:
- Responsibility: Maria shows full responsibility. They initiated the project, led the team, managed resources, and engaged with community leaders and schools to make the project a reality. Alex also ensured continuous improvement of the program based on feedback.
- Complexity: The project is highly complex as it involved setting up a structured program, securing funding, managing a team, and sustaining the initiative over two school years.
- Novelty: Maria’s project demonstrates fully self-initiated novelty. The idea was original, and Maria designed the entire process from concept to execution, filling a specific community need not previously addressed.
Verification Evidence:
- Grant approval letters.
- Testimonials from school administrators.
- Photos and videos from coding sessions.
- Reflective essays written by Alex discussing challenges and successes.
AI Analysis and Credentialing:
The AI tools analyze the submitted evidence and provide a high confidence rating in competencies such as leadership, problem-solving, and community engagement based on the depth and quality of the evidence.
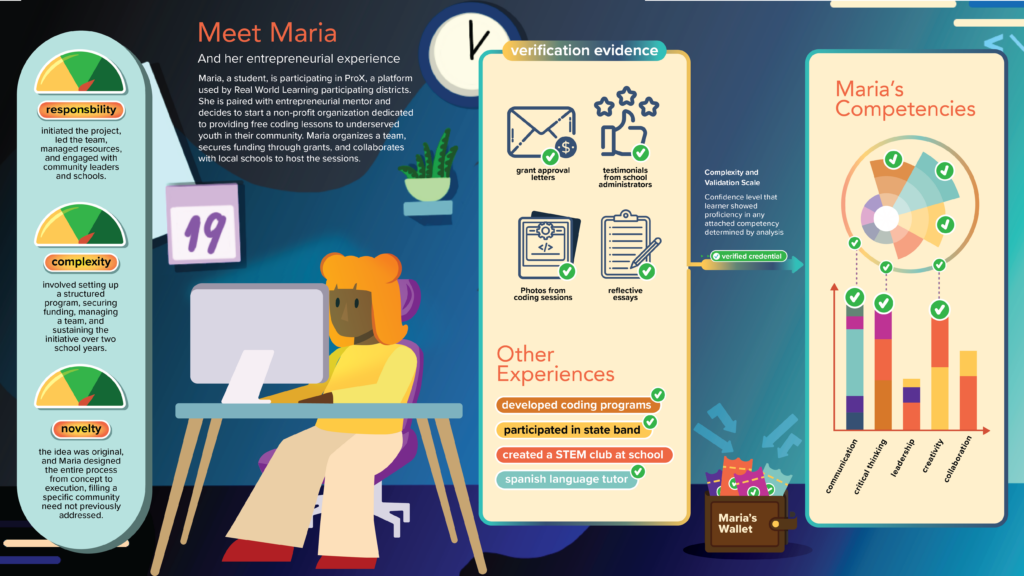
Maria’s Next Steps
- Higher Education Admissions: Based on the confidence rating and the competencies demonstrated, universities interested in students with proven leadership and community service skills highly regard Alex’s application. Alex could receive offers from programs that emphasize social entrepreneurship or community development.
- Employment: Companies looking for young leaders with experience in managing projects and teams might reach out to Alex for intern roles, especially in corporate social responsibility (CSR) sectors.
- Upskilling/Promotion: As Alex continues to develop the non-profit, they might participate in additional training and certification in non-profit management and fund development, further enhancing their credentials and readiness for larger roles.
- Continuous Feedback and Improvement: The credentialing system could suggest areas for further development, such as enhancing financial management skills or expanding the non-profit’s reach, providing a personalized learning and growth roadmap based on the AI’s analysis of skills gaps.